Cnam will host a talk by Professor Badr Youakim from Pennsylvania State University, USA. His talk will be followed by presentations from two Cnam PhD students.
When: November 14, 2024 at 2 pm.
Where: room 35.1.53, 2 rue Conté, CNAM, Paris, France.
Speaker Professor Badr Youakim
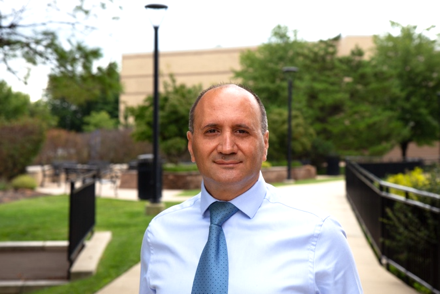
Title: Unveiling the Security Landscape of Agentic AI: From Vulnerabilities to Offensive and Defensive Strategies
Abstract: The rise of Large Language Model (LLM)-based agents, or Agentic AI, marks a significant milestone in artificial intelligence, bringing forth transformative capabilities alongside complex cybersecurity challenges. This talk delves into the intricate world of Agentic AI, focusing on its inherent vulnerabilities and its dual role in shaping offensive and defensive cybersecurity strategies. The talk explores the cybersecurity risks posed by LLM-based agents, including susceptibility to adversarial attacks, data manipulation, and exploitation through prompt injections. Conversely, Agentic AI could be a powerful ally in cybersecurity and play a dual role in shaping offensive and defensive cybersecurity strategies. For example, AI multi-agent systems can be deployed to craft sophisticated attack scenarios to test system robustness and communication networks, thereby aiding in the development of more resilient infrastructures. Join us to uncover how Agentic AI is redefining the boundaries of cybersecurity, presenting new challenges, and offering innovative solutions in the perpetual battle against cyber threats.
Bio: Youakim Badr received the Ph.D. degree in Computer Science from the National Institute of Applied Sciences (INSA-Lyon), France, in 2003. He is a tenured Full Professor of data analytics and artificial Intelligence with the Pennsylvania State University – Great Valley. He holds the position of professor-in-charge for the Master of Artificial Intelligence programs. His wide-ranging academic responsibilities encompass teaching a variety of courses, including foundations of Artificial Intelligence, deep learning, natural language processing, data mining, predictive analytics, and design and implementation of AI-based systems. Dr. Badr’s research is primarily centered on the design and deployment of Trustworthy AI Service Systems. He adopts a comprehensive and interdisciplinary approach, emphasizing Data Centric AI analytics, Trustworthy AI systems, and Composable AI systems. He has had over 140 peer-reviewed publications, including 3 books. Additionally, he fulfills the role of a reviewer for both national and international research funding programs (NSF, ANR, NSERC, Horizon Europe). Dr. Badr is honored with a lifetime membership with ACM and holds an academic associate membership of the Linux Foundation for AI and Data (LFAI&Data).
Speaker: Billal Mokhtari
Title: Cyber-physical security design and integration for interoperability, traceability, and environmental impact reduction in battery cell manufacturing, enhanced by digital twins.
Abstract: My thesis, conducted within the framework of the European BaTTwin project, aims to use ontologies and AI techniques such as Graph Neural Networks (GNN) to detect cyber-physical system attacks in real-time within the battery cell manufacturing chain, based on collected data. The research focuses on identifying security threats and designing interoperability models to integrate cyber-physical security, while exploring approaches based on semantic graphs and even machine learning to improve anomaly detection
Bio: First year PhD student at CNAM, supervised by Samia Bouzefrane and Nada Mimouni.
Speaker: Moheed-Ali KAYANI
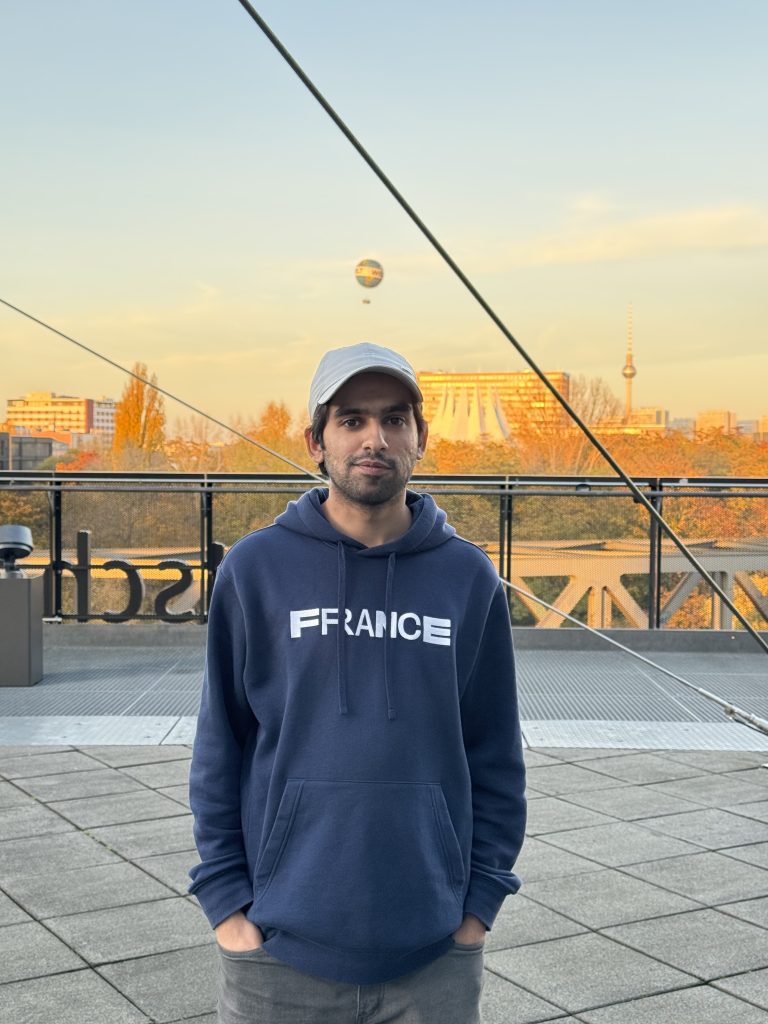
Title: Using AIML approaches for Massive IoT network automation
Abstract: In this research, we aim to leverage AI/ML approaches to enhance automation and optimization in massive IoT networks, particularly as these networks evolve with Beyond 5G and 6G technologies. Our goal is to address the critical performance indicators (KPIs) necessary for IoT applications, including ultra-low latency, high reliability, and energy efficiency, by applying intelligent algorithms that can support real-time decision-making, resource allocation, and anomaly detection. We will explore AI/ML-driven solutions for technologies such as LoRaWAN and vehicular networks, focusing on how these approaches can improve network scalability and adaptability. As 6G networks introduce increasingly complex and demanding requirements, our research will investigate the essential role of AI/ML in meeting KPI expectations, driving intelligent network management, and enabling sustainable, large-scale IoT deployments
Bio: Moheed Ali Kayani is a PhD candidate in the CEDRIC Lab’s ROC team at CNAM, under the supervision of Stefano Secci and Stephane Rovedakis. He received his BSc in Software Engineering from Superior University, Lahore, Pakistan, in the 2018-2022 session, and a Master’s in Computer Networks and IoT Systems from CNAM, Paris, France, in the 2022-2024 session. His research interests include AI/ML approaches in network optimization and automation, Massive IoT networks, LoRaWAN, and vehicular networks. His previous work involves integrating a mobility module into eLoRa (an ns-3 module for end-to-end LoRaWAN emulation) and setting up a testbed to collect data, which he then used with LSTM Autoencoders for anomaly detection.